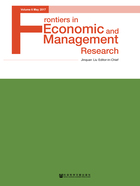
A Study on the Nonlinear Correlation between the Chinese and US Business Cycles and the Monetary Policy Rule
—Empirical Evidence from the Great Moderation, Soft Landing, and Modern Recession Periods
Dayu Liu Tingting Zhao[1]
Abstract: This paper uses the multiple threshold effect test on the monetary policy rules of the Chinese and American monetary authorities during the “Great Moderation”, “Soft Landing” and “Modern Recession” periods to discover a clear asymmetric preference in the adjustment of nominal interest rates. In times of economic expansion, the nominal interest rate adjustments by the two monetary authorities show a preference for avoiding inflation; however, in times of economic contraction, monetary policy is slanted in favor of closing the output gap. Next, this paper uses an LT-TVP-VAR model under the New Keynesian framework to estimate the time-varying parameters for monetary policy rules to discern the effectiveness of the nominal interest rate adjustments by the Federal Reserve during the “Modern Recession” in curbing business cycle fluctuations. Results show that during the “Modern Recession”, monetary policy is unable to affect a counter-cyclical economic expansion solely through nominal interest rate adjustments. Reversing a continued economic slide requires unconventional monetary policy such as Forward Guidance, Operation Twist, and Quantitative Easing, in order to improve economic vitality and stimulate economic growth.
Keywords: Business Cycle; Monetary Policy Rule; Multiple Threshold Model; LT-TVP-VAR Model
1 Introduction
Since the US Subprime Crisis, the causes of the “Great Moderation”, “Soft Landing”, and “Great Recession” have become a focal point of business cycle research. The economic recovery of 1984 marked the start of the “Great Moderation”, a period in which the United States experienced steady economic growth with a great reduction in business cycle fluctuations. During this period, monetary policy controls were also more effective; even though the US experienced recessions in 1990 and 2001, the magnitude of the economic contractions were smaller than in the past. Thus to many economists the US economy had entered a new period of steady growth, which they named the “Great Moderation” (McConnell and Perez-Qurios, 2000; Kim and Nelson, 2004). However, the sudden arrival of the Subprime Crisis gave the US its most severe recession since World War II, and brought a halt to discussion of the “Great Moderation” as economists turned their eyes to the effects of non-cyclical changes on the economy. A “Modern Recession”, as coined by the American economist Hall, is defined as a recession during which monetary policy remains effective and maintains consistency, and the labor market exhibits low friction. Although this definition is insufficient in and of itself to conclude a transition in the US business cycle, it represents a new type of business cycle convergence which is worthy of our attention. Even though it is the longest since World War II, when compared to the “Great Depression” and “Stagflation” in the 70’s, the moderate decline of the “Great Recession” is more in line with those of 1990 and 2001. However, these two recessions were due to rapid increases in the nominal interest rate by the Federal Reserve, but before the “Great Recession”, the Federal Reserve was in the process of “inflation targeting”, and interest rates were not at a high level. Currently, economists lack understanding of such economic recessions without clear causes, and this has become an important area of modern business cycle research.
In comparison to a “Modern Recession”, an economic “Soft Landing” is more clearly defined, specifically as the steady return to moderate economic growth after a period of excessive expansion. Usually, a “Soft Landing” is an ideal slowdown after excessive expansion, since it requires economic growth to remain positive while inflation returns to a suitable range. At this point in time, it is very unlikely for China’s economy to experience negative economic growth, as such the definition for a Chinese “Soft Landing” needs adjustment. In 1997, Chinese M2 year-on-year growth fell to 19.3%, CPI fell to below 4%, and yet GDP maintained a growth rate of over 8%. This shows that China successfully realized its first economic “Soft Landing” since its Economic Reform. For this reason, economists often define a Chinese economic “Soft Landing” as a growth rate of over 7% and inflation rate below 4.5%.
From the definitions of the “Great Moderation”, “Modern Recession” and “Soft Landing”, we see that “Great Moderation” refers to an ideal business cycle, where inflation and volatility remains low and yet monetary policy controls remain effective, whereas “Soft Landing” and “Modern Recession” both refer to two types of cyclical contraction, where economic growth slows after a period of continued expansion. The periods of moderate economic slowdown in the US in 1990 and 2001, as well as the economic landing in China from 1996-2001 all possess characteristics of a “Soft Landing”. However, the US economic slowdown caused by the Subprime Crisis is a classic “Modern Recession”. Thus, we see that even a period of economic stability, such as the “Great Moderation”, can periodically show symptoms of a recession; this is a matter which deserves more of our attention.
In a comparison of the effectiveness of monetary policy during the three periods, “inflation targeting” based monetary policy was able to curb economic volatility during the “Great Moderation” and “Soft Landing” periods, but unable to exert effective control during the “Modern Recession” period (Mayer and Scharler, 2011). During the “Modern Recession”, the Federal Reserve primarily utilized Forward Guidance, Operation Twist, and Quantitative Easing while maintaining a zero-bound interest rate to introduce liquidity and thus affect a counter-cyclical economic expansion. Although economists are currently unable to affirm that unconventional monetary policy operations will be able to pull the US out of its “Modern Recession”, it has been shown that they have been more effective than conventional monetary policy during the current recession (Cúrdia et al., 2015). Data from third quarter of 2014 shows year-on-year real GDP growth in the US reached 2.35%, while inflation returned to a reasonable range, whereas Chinese economic growth fell to 7.3% and inflation remained below 2%. This has brought discussion over whether the US should end its zero-bound interest rate policy and China should loosen monetary policy back into the academic spotlight. A natural question is, are unconventional monetary policy operations able to effectively ease a nation out of a “Modern Recession”? To answer this question, this paper examines the nonlinear correlation between monetary policy rule and the Chinese and US business cycles in different periods. Using the different control targets of different periods, this paper analyzes the causes of the “Great Moderation”, “Soft Landing” and “Modern Recession”, and provides suggestions regarding China’s monetary policy and risk of economic contraction.
2 Theoretical Analysis and Literature Review
2.1 Literature Review
Even though economists have long debated over merits and flaws of discretionary and rule-based monetary policy, empirical studies have shown that the majority of monetary authorities utilize rule-based monetary policy. Early on, Taylor (1993) used a simple linear equation to characterize the reactions of the Federal Reserve’s monetary policy towards economic performance (such as the output gap and inflation rate). The article found that asides from the Federal Reserve’s emergency measures in response to Black Monday (the Stock Market Crash of 1987), this simple linear equation was capable of capturing the Federal Reserve’s monetary policy operations. The “Taylor Rule” not only effectively captures the Fed’s monetary policy operations during the “Great Moderation”, it also laid the foundation for research into rule-based monetary operations. Afterwards, economists began to look into whether “Inflation Targeting” rule-based monetary policy is capable of effectively reducing business cycle volatility. They found that nations that implemented rule-based monetary policy often have relatively low rates of inflation. “Inflation Targeting” monetary policy is able to effectively curb hyperinflation because of its transparency and credibility (Bleier et al., 1999; Faust and Svensson, 2002; Jensen, 2002; Mishkin, 2004). In order to further verify the effectiveness of rule-based monetary policy, Boivin and Giannoni (2006) extended the sample to 1979, and discovered that inflation targeting interest rate rules implemented in 1979 was an important factor behind the “Great Moderation”. During this time, the interest rate was proved extremely effective in controlling inflation; this not only held volatility to a low level, but also prevented a return of “Stagflation”.
Furthermore, many economists have performed research comparing the correlation between monetary policy and the business cycle during the “Great Moderation” in the US and the “Soft Landing” period in China. Research by Clarida et al. (2000), Kim et al. (2004) and Stock and Watson (2005) shows that during the “Great Moderation” period, rule-based monetary policy was able to effectively curb economic volatility; even during periods of cyclical contraction, the magnitude of the contraction was significantly less than in the past (for example, the “Great Depression”, and “Stagflation”). Mayer and Scharler (2011) used sample data in 1959-2009 to re-estimate noise data and interest rate shock; they found that during the “Great Moderation” the US economy did not receive any strong external shocks and concluded that this was the primary reason for the reduction in output volatility. Xie and Luo (2002) used a best response function to test the monetary policy rules of the Chinese monetary authorities; they found that since the “Soft Landing” in 1996, “Taylor Rule” is an accurate fit for monetary policy operations in the period, which shows that rule-based monetary policy served an active role during China’s economic “Soft Landing”. Lu and Zhong (2003) used cointegration analysis to estimate China’s monetary policy rules, finding that “Inflation Targeting” rules not only provide a good fit for interest rate movements, but also forward guidance for monetary policy operations. Zheng and Liu (2010) used a Markov Regime Switching Model with time-varying parameters to re-estimate China’s Taylor Rule, to find that the best response function for monetary policy shows regime switching characteristics. Later, Zhang and Liu (2013) built upon this research, introducing a threshold effect to the Taylor Rule model to estimate China’s interest rate rule; they found that using cumulative real GDP year-on-year growth as the threshold variable, interest rate adjustments show a clear threshold effect with regards to the inflation gap and output gap.
Even though empirical evidence shows Chinese and US monetary authorities followed rule-based monetary policy during the “Great Moderation”, “Soft Landing” and “Modern Recession” periods, there are economists who hold doubts as to whether “inflation targeting” should be the primary rule for monetary policy. Orphanides (2004) and Rabanal (2004) re-estimate the “Taylor Rule” to find that during times of economic contraction, the Federal Reserve adjusts the nominal interest rate primarily according to the output gap. As a result, they believe that rule-based monetary policy cannot simply be ruled the primary cause of the “Great Moderation”; instead it is possible that the reduction in the cyclical output gap and “inflation targeting” interest rate rule only coexisted coincidentally. Furthermore, Bec et al. (2002), Nobay and Peel (2003) and Cukierman and Gerlach (2003) believe that the welfare loss function faced by monetary authorities is different in different periods; in times of economic contraction, interest rate controls are slanted towards closing the output gap. Therefore, after the outbreak of the US Subprime Crisis, the Federal Reserve began using unconventional monetary policy operations such as Forward Guidance, Operation Twist, and Quantitative Easing, resulting in the Federal Funds Rate remaining zero-bound, which in turn eased the effect on “Inflation Targeting” (Gali and Gambetti, 2009). Later studies on the Federal Reserve’s interest rate rules after the Subprime Crisis show that sustained low interest rates are effective in affecting counter-cyclical economic expansion in the course of curbing business cycle volatility; however, whether this will result in long-term welfare costs remains to be seen (Taylor, 2009; Keen, 2013; Baele et al., 2015).
From the literature review, we can see that policy preferences exhibited by monetary authorities in different periods show clear asymmetric characteristics. During times of economic expansion, monetary authorities base interest rate rules on “Inflation Targeting”; in times of economic contraction, authorities more often adjust the nominal interest rate with the goal of closing the output gap. However, there has been little research into the effectiveness of the interest rate in controlling inflation during times of economic contraction. This raises the question, in times of economic contraction, do interest rate adjustments directed at closing the output gap effectively ensure inflation remains at a reasonable level? This paper utilizes a multiple-threshold model and a time-varying parameter vector autoregressive model with latent threshold factors (LT-TVP-VAR) to analyze the nonlinear correlation mechanism between monetary policy, real output and inflation during different stages of the business cycle.
2.2 Theoretical Framework
In order to find the nominal interest rate rule with relation to the output gap and inflation gap, along with the optimal interest rate reaction function, Boinet and Martin (2008) used the double constraint of the aggregate demand curve and aggregate supply curve to minimize the welfare loss function for Central Bank. The aggregate demand and supply functions are defined as follows:


Equation (1) is based on aggregate demand as defined by the Interest-Savings (IS) Curve, where γ represents the adjustment parameter for the output gap with regards to the real interest rate, E represents the expectation operator, Et yt+1 represents output gap forecast for period t+1 in period t, and μDt represents external demand shocks. Equation (2) represents the aggregate supply curve under the New Keynesian framework, where Etπt+1 represents expected inflation, η and κ represent the adjustment parameter for same period inflation with regards to output gap and expected inflation respectively, and μst represents external supply shocks.
In order to better capture the differences in the welfare loss function between different stages, Boinet and Martin (2008) introduced inflation gap and output gap exponents into the welfare loss function; the expanded form is as follows:

where πt,π* and πt,π* represent inflation and the inflation target in period t, yt represents the output gap in period t, Rt and represent the nominal interest rate and equilibrium interest rate in period t, φ and θ/2 represent the reaction parameters for welfare loss function with regards to the output gap and interest rate divergence respectively, and βπ and βy represent the exponent parameters for the inflation gap and output gap respectively. Therefore, by definition, the welfare loss function is nonlinear. απ and αy represent exponential smoothing factors, and their magnitude decides the rate of expansion for the welfare loss function.[2] For better understanding, the values and characteristics of the parameters are provided below.
Table 1 Welfare Loss Function for Monetary Authorities under Different Parameters

In order to further analyze interest rate adjustment preferences of US and Chinese monetary authorities with regards to the output gap and inflation gap during different stages in the business cycle (expansion and contraction), this paper assumes that monetary authorities set the nominal interest rate at the start of each period, only take into account prior inflation and output gaps, and ignore same period interest rate shocks. Under the constraints given by Equations (1) and (2), minimizing the welfare loss function in Equation (3) gives the optimal interest rate rule below:

where φ is the time discount factor. From Equation (4), we obtain the optimal interest rate:

R*t represents the ideal interest rate, where ,
and
Equation (5) is the optimal interest rate rule for monetary authorities with regards to the output gap and inflation gap. When βπ=βy=1 and απ→0, αy→0, it can be understood that G(yt)→1 and G(πt-π*t)→1; in this case Equation (5) devolves into the forecast-based Taylor Rule:

If monetary authorities primarily adjust the nominal interest rate with regards to the output gap and inflation gap ex post facto, then the backward-looking Taylor Rule is given as follows:

This paper expands on the linear Taylor rule given by Equation (7), introducing the Multiple Threshold Model and testing for nonlinear characteristics in the monetary policy rules of US and Chinese monetary authorities.[3] The linear Taylor rule and multiple threshold model are chosen for two main reasons. One, the linear Taylor Rule is able to effectively capture interest rate operations by US and Chinese monetary authorities, and a function of the output gap and inflation gap possesses greater economic significance than a higher order function. Two, the multiple threshold model is capable of discerning both the economic growth and inflation thresholds, dividing the business cycle into “high-growth-high-inflation”, “high-growth-low-inflation”, “low-growth-high-inflation” and “low-growth-low-inflation” regimes; this allows for identification of differences in monetary policy controls during “Modern Recession” (“low-growth-low-inflation” and “Stagflation” (low-growth-high-inflation) periods, and provides better empirical evidence for policy preferences in different stages of the business cycle.
3 Re-Estimation of Nonlinear Taylor Rule for China and the US
3.1 A Taylor Rule Model Accounting for Interest Rate Smoothing
In studying dependencies between the Federal Reserve’s nominal interest rates and economic output and inflation, Clarida et al. (2000) discovered that Equation (7) was too strict to reflect real interest rate operations by monetary authorities. As such, they assumed interest rate operations were adjusted based on the immediate change in economic output and inflation, thus overlooking the desire of monetary authorities for smoothing interest rate changes. In that view, this paper references Wang (2006) to modify Equation (7) as follows:

Here, Rt represents the nominal interest rate, ρ represents the interest rate smoothing factor, ρ∈[0, 1], where a larger value represents smoother adjustments in the nominal interest rate by monetary authorities. Substituting Equation (7) into Equation (8) gives us a Taylor Rule model incorporating the interest rate smoothing factor as follows:

Since both the US and China have not given clear inflation rate targets, this needs to be taken into account before estimating Equation (9). To date, there have been two methods of doing so:
1. Use the Gibbs Sampling MCMC Algorithm to estimate the latent inflation rate (Zheng and Liu, 2010);
2. Estimate by merging the target inflation rate into intercept term (Zhang and Liu, 2013).
Since the focus of this paper lies with performing multiple regime divisions for interest rate rules, and not estimating target interest rates, this paper utilizes the second method. As such, Equation (9) can be simplified as follows:

Equation (10) states the interest rate directly in terms of the output gap and inflation adjustment. β0, β1, β2 represent the intercept term, the interest rate adjustment coefficient with respect to inflation and the adjustment factor with respect to the output gap, where β0=(1-ρ)(α0-βπ*), β1=(1-ρ)β and β2=(1-ρ)λ.
3.2 Application of Multiple Threshold Model to the Expanded Taylor Rule
3.2.1 Data Processing
Much research has show that the nominal interest rate operations have asymmetric preferences at different stages of the business cycle. In times of economic expansion, monetary authorities adjust the nominal interest rate according to the inflation rate. In times of economic contraction, they are more focused on the output gap (Orphanides, 2004; Zhang and Liu, 2013). However, the period of “Stagflation” in the US shows that even in times of economic contraction, inflation can still be high. As such, monetary authorities need to be especially careful in times of economic contraction, and effectively respond to different types of economic contraction such as “Stagflation”, economic “Soft Landing”, and the “Modern Recession”. In view of this, this paper uses the Multiple Threshold Model to characterize interest rate operations by monetary authorities:

Here, Ii(s1, s2), i=1, 2, 3, 4 represents the Indicator Function; when the constraints within the parentheses are met, Ii(s1, s2)=1, otherwise, Ii(s1, s2)=0. s1 and s2 represent threshold variables, where s1 is the first threshold, and s2 is the second threshold.[4] r1, q1, q2 represent corresponding threshold values respectively. As controlling inflation is the primary concern of monetary authorities, this paper uses inflation as the first threshold, and real GDP YoY growth as the second threshold. The remaining variables (β0, β1, β2, ρ, etc.) represent for Taylor Rule coefficients in different regimes.
This paper performs empirical analysis on Chinese data from Q1 1996 to Q4 2014 and US data from Q3 1960 to Q4 2014, with indicators chosen as follows:
(1) Nominal interest rate. This paper uses the 7-day interbank offered rate as a substitute variable for the Chinese nominal interest rate and the Federal Funds Rate for the US nominal interest rate. This is primarily for the following reasons. First, the interbank offered rate and Federal Funds Rate are the most important benchmark rates in their respective financial markets and offer good comparability; second, both are policy tools of their respective monetary authorities, and are strongly correlated with inflation and output; third, both are often used as a benchmark for both the bonds market and interbank lending and are widely applicable.
(2) Inflation rate. In consideration of data comparability, this paper uses the China Consumer Price Index (CPI) and US Consumer Price Index (CPI) in place of the inflation rate. First, we calculate the quarterly CPI according to the arithmetic mean of the China and US CPI provided by the China Economic Information Network (same month YoY=100), and then use πt=(CPIt-100)×100% to calculate the quarterly inflation rate.
(3) Output gap. This paper uses H-P filtering to obtain output gap data. First, using real GDP growth YoY as provided by the National Bureau of Statistics and the nominal GDP in 1996 to calculate real GDP in terms of 1996. Next, we use X12 Seasonal Adjustment to obtain the real GDP_SA series, Yt. Lastly, we use H-P filtering to separate the latent output, obtaining the output gap, yt:


Figure 1 The China and US Nominal Interest Rates, Inflation Rates, Real GDP Growth and Output Gap
3.2.2 Estimating the Multiple Threshold Taylor Rule Model
Before creating a Multiple Threshold Model, we need to first test the threshold effect on the different variables. This is because we should only use a Threshold Model when the threshold variables show a clear threshold effect. As such, this paper references the test method used by Hansen (1996) to test the Linear China and US Taylor Rule Model for threshold effects. First, we performed threshold testing on the first threshold variable, the inflation rate through the following equation:

Here, our original and alternative hypotheses are as follows:

Where θ1=(β0, β1, β2, ρ) and θ2=(β′0, β′1, β′2, ρ′). If the original hypothesis H0 holds true, that means the Taylor Rule Model does not possess a threshold effect. If not, estimating the Taylor Rule with a Threshold Model could substantially improve the fitness. Hansen’s threshold effect test is as follows:

Here, T represents sample size, and
represent the estimated variance of the Linear Taylor Rule Model and Threshold Taylor Rule Model respectively. Let
represent the value of the threshold variable when
is at its minimum, and we obtain:

Here,. Hansen (1996) obtained the asymptotic distribution of F through bootstrapping. However, this method only works if the linear equation does not possess heteroskedasticity. If the China and US Taylor Rule possess heteroskedasticity, then we need to use the Wald statistic shown below for the threshold test:

Here, R represents the constraint; ,
, and
represent explanatory variable matrices, where
is a matrix of estimated coefficients. Same as above, let the Wald statistic reach its maximum at
:

Here, we can again obtain the asymptotic distribution of the Wald statistic through bootstrapping. If the China and US Taylor Rule Models show a notable threshold effect on the inflation rate (the first threshold), we can then use real GDP growth to further divide the sub samples for the two threshold regions. Repeat the above test for Equations (18) and (19):


3.2.3 Estimating the Multiple Threshold Taylor Rules Model for China and the US
Before estimating the Multiple Threshold Taylor Rule Model, this paper first estimates the Linear Taylor Rule Model for China and the US. To account for potential bias in OLS estimates due to the interest rate lag factor, this paper uses the Generalized Method of Moments (GMM) to estimate the Linear Taylor Rule Model, where the instrumental variables are the lag factors for nominal interest rates, inflation rates and output gaps. Next, this paper uses the lag factors for inflation rates and GDP growth as the first and second threshold variables respectively, to re-estimate the Multiple Threshold Taylor Rule Model and measure differences in interest rate manipulation by monetary authorities at different stages of the business cycle.
Table 2 China Multiple Threshold Taylor Rules Model Re-Estimation

Table 2 shows the results of estimating the Taylor Rule Model for China and the US, with the Multiple Threshold Model divided between low-growth-low-inflation, high-growth-low-inflation and hyperinflation. Of these, the hyperinflation regime (πt>9.7) only includes 17 samples, primarily from the “Stagflation” period. The fits for the Taylor Rules in this regime are exceedingly low (R2=0.13) and all variables are unable to refute the original hypothesis at above a 10% significance level. This means that in this regime, the Federal Reserve did not utilize rule-based monetary policy. After removing outliers, the Multiple Threshold Taylor Rule Model shows much better fit in the low-growth-low-inflation and high-growth-low-inflation regimes compared to the linear model, showing the effectiveness of the Multiple Threshold Model. Graph 2-a uses the two threshold variables to divide the US business cycle; light grey area (regime 1) is primarily centered on 1990 and 2001 during periods of economic stagnation/downturn and “Modern Recession”, and the white areas (regime 2) are primarily centered on the “Great Moderation”. By comparing values of parameters for the different regimes, we can see that in times of economic contraction the adjustment coefficient for inflation, β1, is not significant, whereas the adjustment factor for the output gap, β2, is able to refute the original hypothesis with a significance level below 10% and is greater than 0, showing that in times of economic contraction the Federal Reserve adjusts interest rates primarily with regards to the output gap; meaning a negative output gap will lower nominal interest rates, thus stimulating aggregate demand and achieving the counter-cyclical expansionary effect of monetary policy. During times of economic expansion, the inflation coefficient, β1, is able to refute the original hypothesis under a 10% significance level, but the output gap adjustment factor is not significant, showing that in times of economic expansion, the Federal Reserve utilizes a “Inflation Targeting” rule for monetary policy, and does not adjust the nominal interest rate according to the output gap. However, we need to point out that β′1 is less than 1, showing that in times of economic expansion, nominal interest rate adjustments are unable to entirely curb inflationary shocks. As such, if we want to guard against systemic risks, we also need to utilize policy measures such as price controls in concert with interest rate adjustments. Lastly, the interest rate smoothing factor ρ and ρ′ are both able to refute the original hypothesis under a 1% significance level, showing in times of both economic contraction and expansion, the Federal Reserve has a bias towards interest rate smoothing.
In comparison, Table 2 also shows the estimates for China’s Taylor Rule. The Multiple Threshold Model likewise can be divided into 3 regimes of high-growth-low-inflation, low-growth-high-inflation and high-inflation, where the sample fit for the 3 regimes are 0.987, 0.985 and 0.978 respectively and all better than the Linear Taylor Rule Model, showing the Multiple Threshold Model provides better fit. It can also be seen from the estimated coefficients, in the low-growth-low-inflation regime, the output gap adjustment coefficient and interest rate smoothing factor are both highly significant, meaning the monetary authority is primarily exercising monetary policy according to output gap and interest rate smoothing. Compared to the estimates from the linear model, the interest rate smoothing factor estimate is smaller whereas the output gap adjustment factor is larger (0.536<0.879, 0.506>0.121), showing that in times of low-growth-low-inflation, there is less interest in smoothing the interest rate, and the monetary authority will adjust the nominal interest rate primarily according to the output gap. This can be seen as when the output gap diverges negatively (positively) by 1 unit, the monetary authority will lower (raise) interest rates by 0.506 percentage points. In the high-growth-low-inflation regime, estimates for all variables in the model are highly significant, showing that at these times, the monetary authority will balance the inflation rate, output gap, and interest in a smooth interest rate in adjusting the nominal interest rate. Looking at the coefficient estimates, the adjustment coefficient for the output gap is similar to that of the linear model, and that for the inflation rate is much higher (0.127>0.077), showing in times of economic expansion, monetary authorities adjust the interest rate primarily with regards to the inflation rate. Lastly, when macroeconomic conditions are in a state of high inflation, estimates for all the variables are highly significant, and the interest rate smoothing factor is 0.96, far greater than that for the linear model and the other two regimes. This shows that the People’s Bank of China takes great care to maintain a smooth nominal interest rate in times of high inflation to prevent rapid interest rate increases, which may lead to systemic contraction risks.
By comparing the non-linear correlation mechanism between the China and US business cycles and monetary policy in the sample period, we find the following facts. First, the Multiple Threshold Model estimates show both China and the US experienced high inflation; however, the Federal Reserve did not implement rule-based monetary policy, resulting in serious inflation and failure of monetary policy. In comparison, the Chinese monetary authority since the 1996 economic “Soft Landing” has strictly implemented the Taylor Rule and as a result maintained control even in times of high inflation. Second, with regard to preferences of the monetary authorities in different regimes, in times of economic contraction, the Federal Reserve primarily adjusted nominal interest rates according to the output gap, achieving counter-cyclical expansion and curbing economic volatility; in times of economic expansion, nominal interest rates were adjusted primarily with regards to inflation, with an obvious preference towards preventing high inflation; for China, monetary authorities were more careful, and despite showing similar preferences to the Federal Reserve, maintained balance between inflation and the output gap in times of both contraction and expansion, effectively preventing sudden recessions during economic expansion and high inflation during economic contraction. Lastly, looking at the effectiveness of monetary policy during the “Great Moderation”, economic “Soft Landing” and “Modern Recession” periods, the Federal Reserve’s “Inflation Targeting” policy was able to effectively curb macroeconomic volatility during the “Great Moderation”, but during the “Modern Recession” period, the “Zero Lower Bound” constraint on nominal interest rates was unable to curb external inflationary shocks, and adjustments were made primarily through raising aggregate demand to affect the output gap. In comparison, China’s monetary authority’s nominal interest rate adjustments during the “Soft Landing” showed clear preference for avoiding economic contraction, but were still able to effectively regulate inflation, and thus was able to achieve a balanced result.

Figure 2 The Business Cycle Regimes of China and the US
4 A Dynamic Empirical Analysis by LT-TVP-VAR Model
Through the China and US Multiple Threshold Taylor Rule Model results, we can see that Chinese and US monetary authorities have different approaches to adjusting nominal interest rates during the “Great Moderation”, economic “Soft Landing” and “Modern Recession” periods. Since nominal interest rate adjustments are a continuous, dynamic process, in order to further understand the dynamic effect of nominal interest rates on output and inflationary shocks during different stages in the business cycle (low-growth-low-inflation, high-growth-low-inflation, and high-inflation regimes), this paper created a Latent Threshold-Time Varying Parameter-Variable Auto Regression Model (LT-TVP-VAR)[5], to calculate the impulse reaction function for nominal interest rates with regards to real GDP growth and inflationary shocks. This allows us to compare the differences in the dynamic adjustment mechanisms for monetary policy during the “Great Moderation”, economic “Soft Landing” and “Modern Recession” periods.
4.1 Estimating the Parameter for the LT-TVP-VAR Model
Early on, Primiceri (2005) introduced random volatility to the S-VAR model, giving S-VAR model coefficients and co-variance matrices time varying characteristics, and expanded it to a TVP-VAR model, providing an effective avenue for studying time varying reaction relation between macroeconomic variables. Later, many scholars built upon this contribution, finding that compared to the normal VAR mode, TVP-VAR provided much stronger short-term forecasting (Nakajima, 2011). In order to further understand the time-varying relation between nominal interest rates and output growth or inflation since the Federal Reserve’s “Quantitative Easing”, Nakajima and West (2013) created the Latent Threshold Time Varying Parameter Variable Auto Regression Model (LT-TVP-VAR model). This model is able to effectively identify structural changes from reaction mechanisms between variables and reduce error in estimating the covariance matrix, stabilizing estimates from time varying models. In order to better describe this, this paper introduces a standard S-VAR model:

Here, yt is a n×1 dimension observation vector, A represents a n×n dimension simultaneous coefficient matrix, F1......Fs are 1-s order lag coefficient matrices for observation vector Yt, μt is a n×1 dimension structural shock, μt~N(0,∑∑), where:

Let βi=A-1Fi, Xt=Is⊗(yt-1,...,yt-1) (⊗ represents the Kronecker product). Substitute in A and Σ, and Equation (20) can be simplified into:

This paper references Nakajima and West’s (2013) relevant settings, let time varying parameters obey a random walk process with a latent threshold factor:

Here, βt and αt are parameter vectors, ht=log(σ2t). νt, ξt, ζt are random disturbance terms. Equations (20)-(22) describe the classic TVP-VAR model. In order to build a LT-TVP-VAR model that captures the dynamic mechanism used by the Federal Reserve during the “Modern Recession” period for adjusting the nominal interest rate according to economic output and inflationary shock under the “Zero Lower Bound” constraint, this paper defines the latent threshold as follows:


Here, I(.) represents the indicator function (with values of 0 or 1), db and da are the latent threshold levels for the time varying parameter and simultaneous coefficient respectively.[6] Afterwards, this paper uses an expanded Markov Chain Monte Carlo (MCMC) method to estimate the parameters.
4.2 LT-TVP-VAR Based on the New Keynesian Rational Expectations Framework
In this section, we apply a dynamic latent threshold model under the New Keynesian Rational Expectations Framework to better probe nominal interest rate adjustments with regard to output and inflationary shocks during the “Great Moderation” and “Modern Recession” periods. For ease of analysis, we substitute real GDP growth for the output gap series.[7] As such, aggregate demand and supply as described by Equations (1) and (2) can be rewritten as follows:



Here, μDt, μSt, μRt represent aggregate demand shock, aggregate supply shock and monetary policy shock, where μDt and μSt are subject to the AR(1) process: μDt=ρDμDt-1+εDt, μSt=ρSμSt-1+εSt.
ρS and ρD are the corresponding first stage auto regression coefficients, εS and εD represent random interference terms (εS and εD are uncorrelated). Equations (25)-(27) establish the New Keynesian Monetary Policy Rule Model under the rational expectations framework. If monetary authorities primarily adjust nominal interest rates with respect to real GDP growth and inflation levels after the fact, we can use Equations (25)-(27) to represent this a matrix form with time varying coefficients and latent thresholds as follows:

Among these, Yt=(yt, πt, Rt), μt=(μDt, μSt, μRt), εt=(εDt, εSt, εRt).

This paper uses the MCMC method together with the New Keynesian Monetary Policy Rules to estimate the time varying parameters. In order to ensure stable estimates, we run the MCMC simulation 20,000 times (of which 2000 are empirical samples), with a lag order of 1. Results can be seen in Table 3. Table 3 gives the estimated parameters for the China and US Latent Threshold Time Varying Parameter Monetary Policy Rules. Here, Inef represents effective factors, as defined below:

where, let Bm=500, ρs represent the s order autocorrelation coefficient, and ineffective factors can be used to test the effectiveness of the MCMC simulation. Table 3 shows, most variables have a ineffective factors of less than 200, meaning the MCMC simulation exhibits good convergence. In addition, asides from μa, all variables can refute the original hypothesis below a 10% significance level, meaning the estimated parameters are effective. With regard to latent threshold factor results, dβ for both China and the US has an acceptable rate of greater than 70%, showing the Monetary Policy Rule Equation exhibits a significant threshold effect; the US dα achieves an acceptance rate of 88.8%, but China’s dα achieves only 49.6%, meaning within the sample, the Federal Reserve’s nominal interest rate adjustment volatility is relatively large and possesses obvious structural shocks, whereas China’s monetary authority’s interest rate adjustment is more smooth, and its volatility does not possess a significant threshold effect. For ease of analysis, we provide trend charts for the GDP growth adjustment coefficient, inflation adjustment coefficient and interest rate smoothing factor for the Time Varying Parameter Monetary Policy Rule Equation.
Table 3 Parameter Estimates for Latent Threshold Factor Time-Varying Coefficients


Figure 3 Time Varying Coefficient Path
Figures 3a to 3f show the trends in time varying parameters monetary policy rule equation for China and the US. From Figures 3d to 3f, we can see for China, the trend is stable for both the inflation adjustment coefficient and interest rate smoothing factor within the sample, meaning China’s monetary authority greatly emphasizes smooth interest rates and strictly executes a “target inflation rate” rule; in comparison, the GDP growth adjustment coefficient starts at a high level and slowly falls, with a steady rebounding trend after the “Subprime Crisis”. This is because the beginning of the sample (1996-2001) represents the first economic “Soft Landing” period after China’s market economy reforms. In order to mitigate structural inflation caused by continuous easing, the People’s Bank of China once implemented nominal interest rates higher than 10%. Once inflation returned to rational levels, the monetary authority’s nominal interest rate adjustments gradually tilted towards GDP growth, resulting in the GDP growth adjustment factor remaining at a high level throughout this period. Starting in 2002, China’s macro-economy underwent a new round of “Soft Expansion”, with GDP growth maintaining a level above 10%; the monetary authority gradually moved towards emphasizing controlling inflation during the period of time. From 2012 onwards, with structural economic adjustments and a slowing of stimulus, macroeconomic growth dropped below 8%, and still remains in a continuous contraction period; this tilted nominal interest rate adjustments back towards economic growth. The above analysis shows, in the process of adjusting the nominal interest rate, China’s monetary authority greatly emphasizes the “Inflation Targeting” rule and interest rate smoothness. Beyond this, in times of economic prosperity, nominal interest rates will be less focused on economic growth, yet in times of contraction, emphasis will shift back towards growth.
In comparison, nominal interest rate adjustments by the Federal Reserve show much greater discretion; asides from the stable interest rate smoothing factor, output growth and inflation adjustment coefficients vary significantly over time. At the start of the sample period, the inflation adjustment coefficient started at a high level of 0.15, but as the US economy entered the “Great Moderation”, it gradually fell, stabilizing at a low level. In reality, before the “Great Moderation”, the US economy experienced two rounds of high inflation; during these periods, in order to prevent serious inflation from introducing systemic contraction risks, the Federal Reserve often sharply increased the nominal interest rate, resulting in this period showing a high inflation adjustment coefficient throughout. Since the 1980’s, in order to prevent a reoccurrence of “Stagflation”, the Federal Reserve started implementing a “Inflation Targeting” rule as the basis for monetary policy, which welcomed the “Great Moderation” period. This period left the Federal Reserve well versed as to the importance of maintaining a low Federal Funds Rate, resulting in the inflation adjustment coefficient to gradually stabilize. In contrast to the inflation adjustment coefficient, the output growth coefficient showed much greater counter-cyclical characteristics, with two peaks during the “Oil Crisis” and the 1990’s recession, but a continuous low level throughout the “Great Moderation”. This shows that the Federal Reserve exhibits significant asymmetric tendencies during different stages of the business cycle; during economic expansion, the output growth adjustment coefficient is low, and in times of contraction nominal interest rate adjustments will slant towards growth. In addition, since the Subprime Crisis, the Federal Reserve has already dropped the nominal interest rate to near 0, and the limitations on the rate cause the output growth adjustment factor to significantly decrease. This shows that traditional monetary policy is already unable to curb the effects of the “Modern Recession” on output and as a result, the Federal Reserve started utilizing non-standard monetary policies such as Forward Guidance, Operation Twist and Quantitative Easing to introduce liquidity and stimulate the economy and achieve the counter-cyclical expansion.
Comparing the Time Varying Parameter Monetary Policy Rules Equation for China and the US, we can see: First of all, with regards to the real output growth adjustment coefficient, nominal interest rate adjustments targeting output growth by monetary authorities in both China and the US show clear counter-cyclical characteristics; in times of expansion, output growth adjustment coefficients remain low, and in times of contraction, nominal interest rates will tilt towards supporting output growth. Secondly, looking at the trend in inflation adjustment coefficients, China’s monetary authority greatly emphasizes the “target inflation rate”, and the adjustment coefficient shows little time varying characteristics; however, the Federal Reserve shows much more discretion, specifically during the “Oil Crisis” and “Stagflation”, the inflation adjustment coefficient was high, and since entering the “Great Moderation”, the Federal Reserve began maintaining a low Federal Funds Rate long-term, and the coefficient has stabilized at a low level. Lastly, comparing the effectiveness of traditional monetary policy and non-standard monetary policy, traditional monetary policy was effective in curbing inflation and economic volatility during the “Soft Landing” and “Great Moderation”, however, under the constraint of “Zero Lower Bound”, it was unable to curb the output shock of the “Modern Recession”. As such, the Federal Reserve began implementing Forward Guidance, Operation Twist and Quantitative Easing, which achieved the goal of counter-cyclical expansion, and realized growth and steady inflation in the current business cycle.
5 Conclusions
Even though our understanding of the non-linear correlation between the business cycle and monetary policy is already mature, there is little research on the difference in rules-based monetary policy between countries utilizing the non-linear monetary policy framework with regard to classic periods such as the “Great Moderation”, “Soft Landing” and “Modern Recession”. This paper does just this, dynamically observing the effectiveness of monetary policy tools (such as interest rates and money supply) in regulating target variables (such as output and inflation), and comparing monetary policy preferences and effectiveness at different stages in the business cycle. Actually, the “Modern Recession” period holds many implications, primarily that when a monetary authority is faced with a “Zero Lower Bound” constraint, merely adjusting the nominal interest rate according to predetermined rules and target variables is no longer effective for curbing volatility in the business cycle. As such, this paper introduces a latent threshold time varying parameter model, testing monetary policy rules for non-linear characteristics, while also effectively measuring the dynamic reaction mechanisms of monetary policy during different stages of the business cycle, thus filling in what was missing from this field of research.
This paper first expands upon related research by Boinet and Martin (2008), deriving hindsight optimized monetary policy rules, and utilizing a multiple threshold model to test China and US monetary policy rules from different periods. Our results show within the sample period, China and the US show structural changes in monetary policy, which can be divided into the 3 regimes of low-growth-low-inflation, high-growth-low-inflation and high-inflation. With regards to monetary policy preferences, nominal interest rate adjustments by monetary authorities in both countries tended towards inflation control in times of growth and stimulating growth in times of contraction. However, from an effectiveness standpoint, nominal interest rate adjustments by the monetary authority in China maintained effectiveness throughout the sample period, whereas the Federal Reserve temporarily lost control during “Stagflation” and the “Oil Crisis”. In addition, the “Modern Recession” period illustrates that when nominal interest rates are already near the “Zero Lower Bound”, rule-based monetary policy is unable to effectively curb inflation and output shocks; at this time in order to achieve the counter-cyclical expansion that is the goal of monetary policy, non-standard monetary policy such as Forward Guidance, Operation Twist and Quantitative Easing must be implemented. Next, in order to better identify dynamic characteristics of nominal interest rate adjustments by China and US monetary authorities during the “Great Moderation”, “Soft Landing” and “Modern Recession”, this paper builds a Latent Threshold Time Varying Parameter Monetary Policy Rules Equation on the framework of rational expectations, and utilized the LT-TVP-VAR model to test the dynamic reaction mechanisms for nominal interest rate adjustments with regard to output growth and inflationary shocks by China and US monetary authorities. Our results show the Federal Reserve nominal interest rate adjustments show strong discretionary characteristics with regard to output growth; in times of contraction, adjustments tilt toward the output gap; in times of expansion, nominal interest rate adjustments tend more towards inflation control. China’s monetary authority will also change monetary policy operations in response to the business cycle, but compared to the Federal Reserve, China’s monetary authority puts much more emphasis on smooth interest rates.
In fact, through examining nominal interest rate adjustments by China and US monetary authorities during the “Great Moderation”, the “Soft Landing” and “Modern Recession” periods, we find that at different stages of the business cycle, there is a significant difference in how the two implement nominal interest rate in response to output and inflation shocks. During the “Great Moderation” and “Soft Landing”, nominal interest rate adjustments were able to effectively curb output and inflation shocks, however, during the “Modern Recession” period, regulating effects of traditional monetary policy were extremely limited. Currently, China’s economy has stepped into a new recession period, the balance of growth, prices, interest rates and other macroeconomic variables are quietly changing, and macroeconomic operations have already exhibited periodic characteristics and the “New Normal”. As such, the government and monetary authorities need to proactively adapt to the “New Normal” and pay more attention to the experiences of the nominal interest rate adjustment during the “Great Moderation” and the “Modern Recession”.
References
Baele, Lieven, et al., “Macroeconomic Regimes,” Journal of Monetary Economics 70 (2015): 51-71.
Bec, Frédérique, M. B. Salem, and F. Collard, “Asymmetries in Monetary Policy Reaction Function: Evidence for the U.S. French and German Central Banks,” Studies in Nonlinear Dynamics and Econometrics 6 (2002): 1-22.
Boinet, Virginie, and C. Martin, “Targets, Zones, and Asymmetries: a Flexible Nonlinear Model of Recent UK Monetary Policy,” Oxford Economic Papers 60 (3) (2008):423-439.
Boivin Jean, and M. P. Giannoni, “Has Monetary Policy Become More Effective?” The Review of Economics and Statistics 88 (3) (2006): 445-462.
Camacho, Maximo, G. P. Quiros, and H. R. Mendizabal, “High-Growth Recoveries, Inventories and the Great Moderation,” Journal of Economic Dynamics & Control 35 (2011): 1322-1339.
Chib, Siddhartha, F. Nardari, and N. Shephard, “Markov Chain Monte Carlo Methods for Stochastic Volatility Models,” Journal of Econometrics 108 (2) (2002): 281-316.
Clarida, Richard, J. Galí, and M. Gertler, “Monetary Policy Rules and Macroeconomic Stability: Evidence and Some Theory,” Quarterly Journal of Economics 115 (2000): 147-180.
Cukierman, Alex, and S. Gerlach, “The Inflation Bias Revised: Theory and Some International Evidence,” The Manchester School 71 (2003): 541-565.
Cúrdia, Vasco, et al., “Has U.S. Monetary Policy Tracked the Efficient Interest Rate?” Journal of Monetary Economics 70 (2015): 72-83.
Faust, Jon, and L. E. O. Svensson, “Transparency and Credibility: Monetary Policy with Unobservable Goals,” International Economic Review 42 (2001): 369-397.
Galí, Jordi, and L. Gambetti, “On the Sources of the Great Moderation,” American Economic Journal: Macroeconomics 1 (1)(2009): 26-57.
Hansen, Bruce E., “Inference when a Nuisance Parameter is not Identified under the Null Hypothesis,” Econometrica 64(1996): 413-430.
Jensen, Henrik, “Optimal Degrees of Transparency in Monetary Policy Making,” Scandinavian Journal of Economics 3(2002): 399-422.
Keen, Steve, “A Monetary Minsky Model of the Great Moderation and the Great Recession,” Journal of Economic Behavior &Organization 86(2013): 221-235.
Kim, Chang-Jin, C. R. Nelson, and J. Piger, “The Less-volatile US Economy: a Bayesian Investigation of Timing, Breadth, and Potential Explanations,” Journal of Business & Economic Statistics 22 (1)(2004): 80-93.
Lu, Jun, and D. Zhong, “Cointegration Test of Taylor Rule in China,” The Journal of the World Economy 5(2003): 76-85.
Mayer, Eric, and J. Scharler, “Noisy Information, Interest Rate Shocks and the Great Moderation,” Journal of Macroeconomics 33(2011): 568-581.
McConnell, Margaret M., and G. Perez-Quiros, “Output Fluctuations in the United States: What has Changed since the Early 1980’s?” American Economic Review 90 (5)(2000): 1464-1476.
Mishkin, Frederic S., “Can Inflation Targeting Work in Emerging Market Countries?” NBER Working Paper 2004, No.10646.
Nakajima, Jouchi, and M. West, “Bayesian Analysis of Latent Threshold Dynamic Models,” Journal of Business and Economic Statistics 2(2013): 151-164.
Nakajima, Jouchi, M. Kasuya, and T. Watanabe, “Bayesian Analysis of Time-varying Parameter Vector Autoregressive Model for the Japanese Economy and Monetary Policy,” Journal of the Japanese and International Economics 25 (3) (2011): 225-245.
Nobay, A. Robert, and David A. Peel, “Optimal Discretionary Monetary Policy in a Model of Asymmetric Central Bank Preferences,” Economic Journal 113(2003): 657-665.
Orphanides, Athanasios, “Monetary Policy Rules, Macroeconomic Stability, and Inflation: a View from the Trenches,” Journal of Money, Credit and Banking 36 (2)(2004): 151-175.
Primiceri, Giorgio E, “Time Varying Structural Vector Autoregressions and Monetary Policy,” Review of Economic Studies 3(2005): 821-852.
Rabanal, Pau, “Monetary Policy Rules and the US Business Cycle: Evidence and Implications,” IMF Working Paper 2004, No.164.
Stock James H., and M. W. Watson, “Understanding Changes in International Business Cycle Dynamics,” Journal of the European Economic Association 3 (5)(2005): 968-1006.
Svensson, Lars. E. O., “Inflation Targeting: Should it be Modeled as an Instrument Rule or a Targeting Rule?” European Economic Review 46 (4)(2002):771-780.
Taylor, John B., “Discretion Versus Policy Rules in Practice,” Carnegie-Rochester Conference Series on Public Policy 39(1993): 195-214.
Taylor, John B., “The Financial Crisis and the Policy Responses: an Empirical Analysis of What Went Wrong,” NBER Working Paper 2009, No.14631.
Wang, Jianguo, “An Empirical Study on the Taylor Rule and China’s Monetary Policy Reaction Function,” The Journal of Quantitative & Technical Economics 1(2006):43-49.
Xie Ping, and X. Luo, “Taylor Rule and Its Empirical Test in China’s Monetary Policy,” Economic Research Journal 3(2002): 3-12.
Zhang, Xiaoyu, and J. Q. Liu, “A Study on the Non-linear Correlation Mechanism between Ruled Monetary Policy and the Business Cycle,” The Journal of World Economy 11(2013): 3-26.
Zheng, Tingguo, and J. Q. Liu, “Taylor Rule with Regime Switching and Its Application to China’s Monetary Policy,” Economic Research Journal 3(2010): 40-52.
[1] Dayu Liu, Center for Quantitative Economics of Jilin University; Tingting Zhao, Statistics Department in Columbia.
[2] Here, let βπ and βy be 1, 2 and 3. When βπ=βy=1 and απ→0, αy→0, the welfare loss function in Equation (3) becomes a second order function. Relaxing the assumptions απ→0, αy→0, it becomes linear exponential and α determines the direction of asymmetry (when α>0, welfare loss is directly correlated with the direction of the inflation/output gaps, and vice versa). If the assumption βπ(βπ)=1 is also relaxed, the welfare function in Equation (3) exhibits a passive region. Specifically, when βπ(βπ)=2, said region is symmetric, and when βπ(βπ)=3, it is asymmetric. For more information, see Boinet and Martin (2008).
[3] We performed estimations under 9 different sets of conditions for βπ, βy, and discovered that the Linear Taylor Rules (βπ=βy=1) exhibited the best fit for both China and the US. If interested, please contact the author for relevant data and estimation results.
[4] This paper uses sequential thresholds. When applying the second threshold to the regimes divided by the first threshold, it is applied independently to the 2 sub regions, which means the second threshold has two threshold values, q1 and q2, one for each sub region. This type of sequential threshold model holds great economic significance, as economic growth desired by monetary authorities is significantly different during different stages of the business cycle.
[5] The primary reason we chose the LT-TVP-VAR model to analyze time-varying mechanisms for monetary policy is after the Federal Reserve implemented its “Zero Lower Bound” and related non-standard monetary policies, the nominal interest rate underwent a structural change. Estimating time-varying parameters using just a TVP-VAR model with random fluctuations would magnify shocks in times of structural change, thereby increasing the estimation error for the covariance matrix. The LT-TVP-VAR model avoids this by introducing time-varying coefficients for latent threshold variables.
[6] Specifically, when latent threshold factor db=da=0, LT-TVP-VAR regresses to a TVP-VAR model.
[7] There were two main reasons for substituting real GDP growth for output gap. 1, dynamic analysis has greater requirements for data accuracy, and the output gap is an estimated value not suited for dynamic analysis; H-P filter, B-P filter and even the production function method all have elevated error at their endpoints of a period. 2, in adjusting the nominal interest rate, monetary authorities treat real GDP growth as an important reference point.